Authors:
Anders Dalgaard and Vincent Laulagnet. Anders, an Executive MBA from the Technical University of Denmark (DTU), has years of experience working with customer 360 advanced analytics and customer journeys. Vincent, a generative AI developer, has implemented analytics to handle and analyse customer complaints for large pharmaceutical companies.
Abstract:
Customer service recovery is a critical business function with vast potential for innovation and competitive advantage. Generative AI offers transformative possibilities in this domain, but simply deploying chatbots is insufficient. We advocate a strategic approach where businesses first leverage generative AI and analytics to comprehensively understand customer journeys, leading to more meaningful interactions and targeted interventions. This foundation enables effective automation and a superior customer experience.
Introduction:
Everyone makes mistakes—both individuals and businesses. How you handle and learn from these mistakes and service failures truly defines you. The Service Recovery Paradox is a crucial and often undervalued component of customer service, as it can transform a negative experience into a positive one, fostering loyalty, retention, and, therefore, a higher Customer Lifetime Value. Research from the Customer Service Institute of America (see link ) highlights how effective service recovery can reduce customer churn, enhance brand reputation, and boost profitability. Obviously, no one should make mistakes on purpose. However, reported service failures (i.e., complaints) are likely to occur. If dealt with appropriately, these are valuable sources of outside-in knowledge, development inspiration, and accelerators to increase customer loyalty even higher than if no service failure had happened (as illustrated below). There is also a healthy mental model to remind ourselves that loyal customers complain, and the rest of the dissatisfied customers leave you quietly.
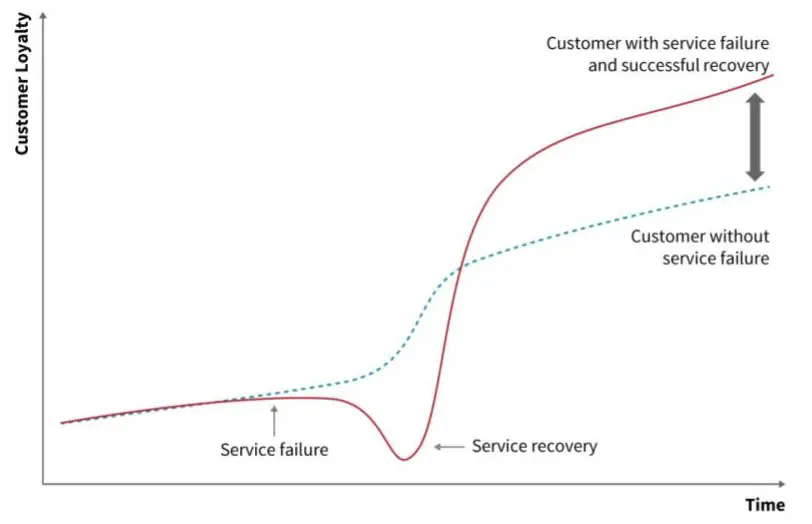
Source: McCollough & Bharadwaj (1992)
So, in reality, it is a powerful company differentiator opportunity in a competitive market in terms of how you prepare your Customer Service department, your Call Center, etc., to deal with service failures. This is where granular data-driven insights per customer ID across channels and touch points over time impact individual treatment. Here, we seek a balanced positive insubordination paradigm to achieve the goal in the illustration below. At all times, we need to understand better the customers’ Jobs To Be Done to get the proper context and, therefore, a meaningful resolution given the service failure.
All this touches and often changes the company culture, values, the level of decentralisation of discretionary authorisations, processes, IT support, applied analytics, leadership style, skills (hard and soft), KPIs, potentially how you are organised, and much more. It is not just a tech or Generative AI thing. This is, after all, a people thing—at least until smart chatbots 100% can take over this domain as well at some point in time.
Industry analysts recognise the potential of generative AI to revolutionise customer interactions. Imagine AI instantly summarising feedback across multiple channels, analysing sentiment, and pinpointing recurring issues. We envision AI crafting personalised responses to inquiries tailored to individual preferences and past history. These are just a glimpse of how generative AI can elevate customer service and recovery.
Scaling with a Strategic Approach:
While generative AI chatbots offer scalability, rushing their deployment can exacerbate existing problems. Chatbots trained on outdated data won’t deliver the desired outcomes. Moreover, automation alone doesn’t provide the insights needed for service and product improvement. Some customers may even prefer other communication channels.
We propose an alternative: use generative AI and analytics to develop a deep understanding of your customers. This involves accelerating issue resolution, uncovering root causes, and designing tailored support and engagement solutions. The six steps are illustrated below:
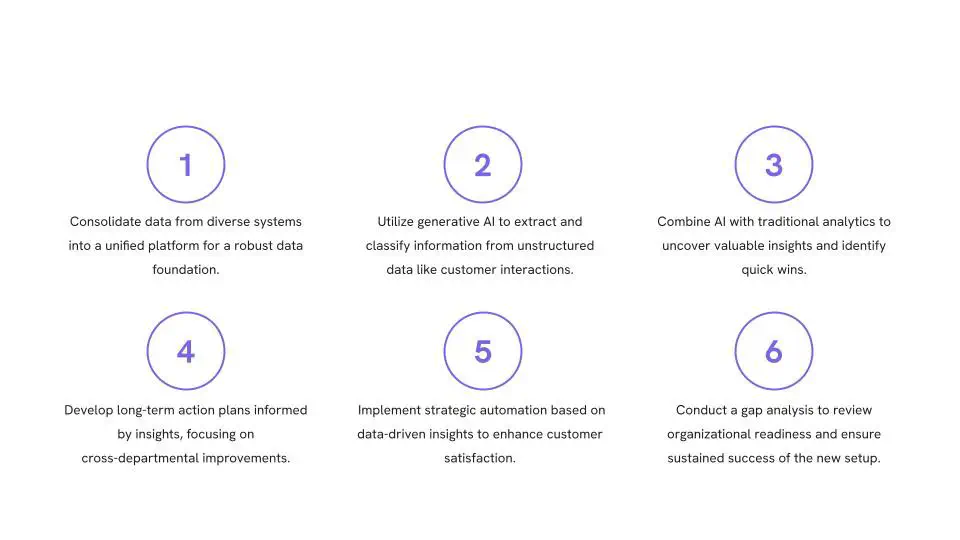
Step 1: Building a Robust Data Foundation: Start by selecting concrete use cases that align with your business objectives and customer needs. Once these use cases are clearly defined and prioritised, identify the required data for each. This data should encompass all aspects necessary to address the specific Jobs To be Done. Next, consolidate data from diverse systems, such as customer support, issue management, and call centers, into a unified platform. Integrating this data will create a comprehensive and cohesive data foundation that supports practical analysis and insights.
Step 2: Enriching your data with Generative AI: Generative AI can accelerate and enhance your data foundation by extracting information from unstructured data like customer interactions, identifying complaints, classifying their nature, assessing severity, and even tracking troubleshooting steps. This yields richer, more comprehensive data than traditional methods.
Step 3: Gaining Insights and Implementing: Quick Wins combining generative AI with traditional analytics to uncover valuable insights. Identify gaps in support documentation, pinpoint staff training needs, or discover product defect patterns. These insights enable quick fixes that boost customer satisfaction and prevent future issues.
Step 4: Defining Actions and Continuous Monitoring: Beyond quick fixes, insights can inform comprehensive, long-term action plans. These may involve multiple departments and focus on improving products, services, or processes. Monitoring the impact of these actions is vital for continuous improvement.
Step 5: Strategic Automation: With data-driven insights and monitoring in place, automate specific tasks strategically. Use AI to generate personalised responses, route inquiries, or proactively empathetically address and resolve potential problems (service failures). By grounding automation in a deep understanding of customer needs (Jobs To Be Done), you maximise its effectiveness, and you give the customer representative/call centre agent/sales person meaningful remedy options to choose from in optimising the expected business outcomes over time.
Step 6: Review the organisation’s current readiness: A gap analysis will show how to embed and adopt this new Service Recovery setup in the organisation to ensure its likelihood of sustained success.
Conclusion:
Generative AI has the power to transform how businesses interact with customers. But automation is just one part of the equation. The true potential lies in using AI to accelerate data collection and analysis, unlocking deeper insights and driving more effective action. Early adopters who embrace this holistic approach will secure a substantial competitive advantage over time, and, like so many other initiatives, Service Recovery is a multi-faceted change management effort worth undertaking. We would love to hear your thoughts and comments on our suggested approach and discuss how it can address your organisation’s challenges